Making Critical, Ethical Software
Machine learning is a large and important part of product design. It’s in most everyday things that users touch–from Google’s search results, to internet ads, to Netflix recommendations. Machine learning algorithms have also been used in biased and unjust prison sentencing, in facial detection (or lack thereof), in biased hiring practices that favor male applicants in technical roles, and in other ways that harm everyday people in everyday ways. Technology can amplify harm, bias, misogyny, racism, and white supremacy. Even if technology cannot create equality, technology as a field needs to be examined and remade in order to create some spaces of equity.
What would it look like to create technology that acts as harm reduction, that acts actively as critique, as an artistic as well as a technical intervention? Where are the intersections of machine learning with tech and with art? Tania Bruguera’s concept of “Arte Útil,” or “Useful Art,” explores this notion of usefulness, of utilitarianism, to create an intervention that is both an artwork and a tool.
Useful Art is a way of working with aesthetic experiences that focus on the implementation of art in society where art’s function is no longer to be a space for “signaling” problems, but the place from which to create the proposal and implementation of possible solutions. We should go back to the times when art was not something to look at in awe, but something to generate from. If it is political art, it deals with the consequences, if it deals with the consequences, I think it has to be useful art.[1]
Feminist Data Set started in 2017 as a response to the many documented cases of problems in technology and bias in machine learning. It is a critical research and art project that examines bias in machine learning through data collection, data training, neural networks, and new forms of user interface (UI), as well as an the projected creation of a feminist artificial intelligence (AI). Inspired by the work of the maker movement, critical design, Arte Útil, the Critical Engineering Manifesto, Xenofeminism, and the Feminist Principles of the Internet, Feminist Data Set is a multi-year project designed to counteract bias in data and machine learning.
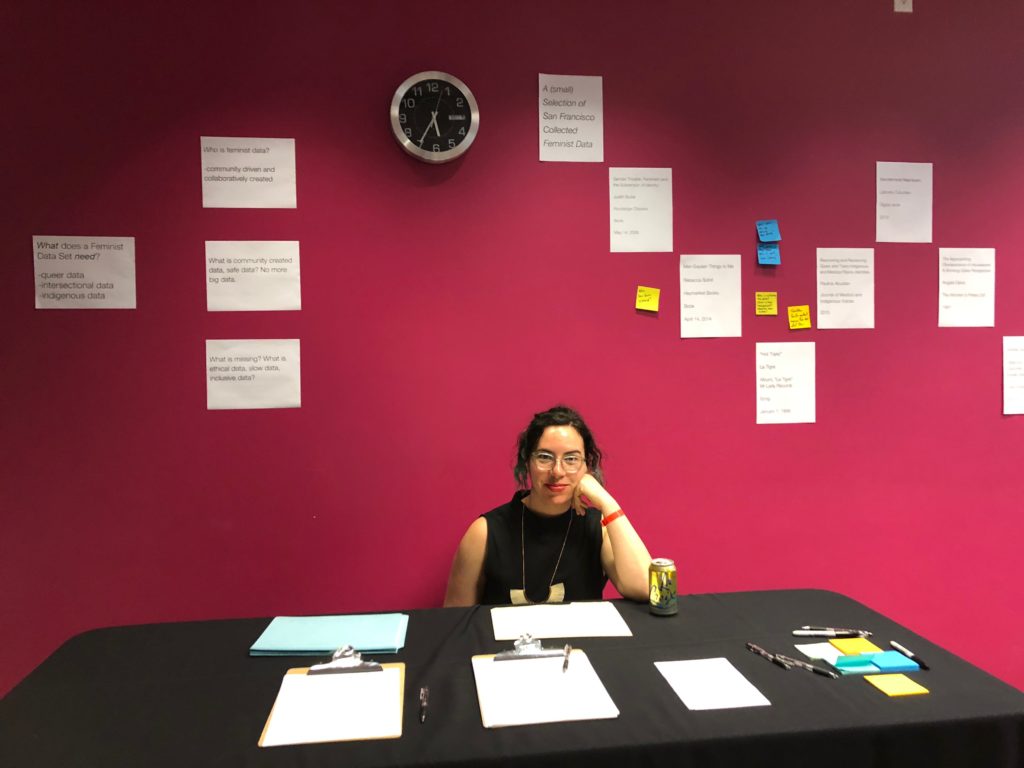
Image of the artist holding an in person performance of active data collection for Feminist Data Set for Yerba Buena Center for the Arts “Public Square” event (image courtesy of the artist, Caroline Sinders).
In machine learning, data is what defines the algorithm: it determines what the algorithm does. In this way, data is activated: it has a particular purpose, it is as important as the code of the algorithm. But so many algorithms exist as proprietary software, as black boxes that are impossible to unpack. For instance, why does Facebook’s timeline serve up certain posts at certain times by certain users? Why do you see some friends’ photos more than others? Facebook’s timeline is driven by a black box algorithm designed to give you selective and “important” content; these standards of “important” are determined by Facebook and are not shared with the general public, nor are they open to public critique or feedback. How Facebook responds and processes a user’s data is considered proprietary; as a user, you do not own your data, Facebook does.
Data inside of software, and especially in social networks, comes from people. What someone likes, when they talk to friends, and how they use a platform is human data—it’s never cold, mechanical, or benign. Data inside of social networks is intimate data, because conversations and social interactions, be they IRL or online, are varying forms of intimacy. How people interact with each other are and what they like, and post, and dislike are “things” that are completely human; those “things” are also data.
Feminist Data Set imagines data creation, as well as data sets and archiving, as an act of protest. In a time where so much personal data is caught and hidden by large technology companies, used for targeted advertising and algorithmic suggestions, what does it mean to make a data set about political ideology, one designed for use as protest? How can data sets come from creative spaces, how can they be communal acts and works? What are the possibilities of a data set about a community that is made by that community? It can be a self-portrait, it can be protest, it can be a demand to be seen, it can be intervention or confrontation. It can be incredibly political. What about how a system then interprets that data? What if that system were also open to critique as well as community input?
Ethical, communal, “hackable” design and technology is a start towards an equitable future. It allows for community input, and for a community to drive or change a decision about a product, its technical capability, and its infrastructure. Feminist Principles of the Internet, a manifesto that addresses how to build feminist technology for the internet, builds upon this ethos by recognizing that in the process of making technical infrastructure, who it’s built for has political ramifications. Feminist Principles of the Internet pushes open source technology and communities further by demanding space for marginalized groups and intent within technology, and it’s this ethos in which Feminist Data Set exists.
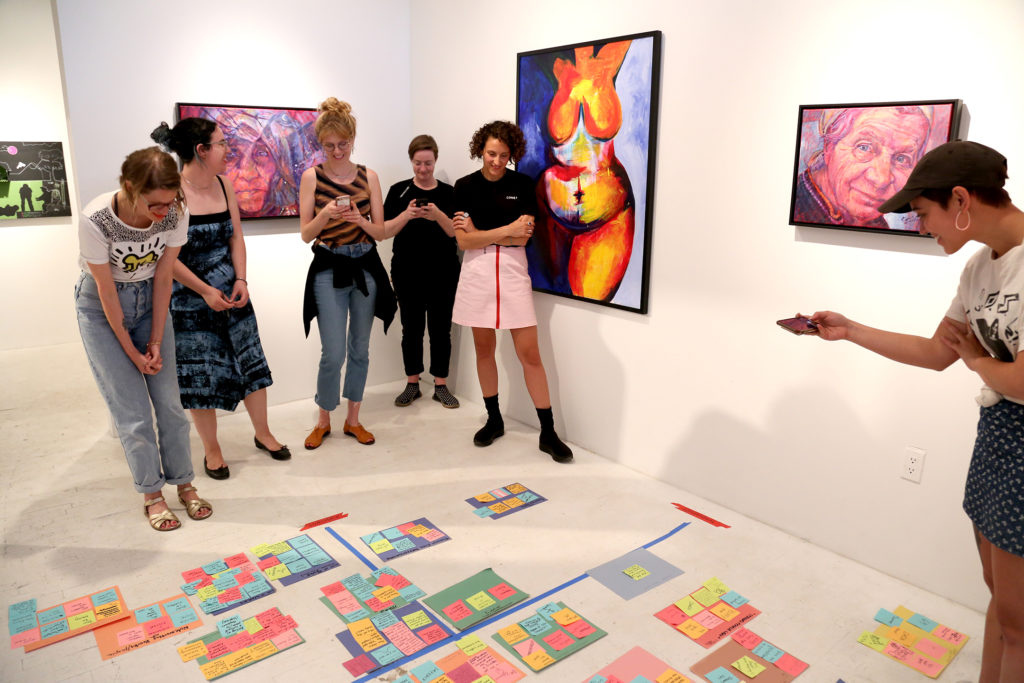
Image of the artist holding a workshop to create the data taxonomy and matrix for Feminist Data Set (image courtesy of SOHO20 gallery).
Feminist Principles of the Internet, as well as theories like cyborg feminism and Xenofeminism, calls for a change in technology and how it functions, as well as a change of leadership and ownership for that technology. The manifesto of Feminist Principles of the Internet demands a redefinition and re-purposing of technology and open source: “Women and queer persons have the right to code, design, adapt and critically and sustainably use ICTs and reclaim technology as a platform for creativity and expression, as well as to challenge the cultures of sexism and discrimination in all spaces.”[2] Within this document, Feminist Principles of the Internet defines “agency” as a necessary form of empowerment. “We call on the need to build an ethics and politics of consent into the culture, design, policies and terms of service of internet platforms. Women’s agency lies in their ability to make informed decisions on what aspects of their public or private lives to share online.”[3] Feminist Data Set exists within those realms of both technology and agency, as a critique on current machine learning infrastructure and practices, as well as a technical framework, critical methodology, and practice-based artwork attempting to address these issues.
This kind of re-examining of what software can do, and should do, is similar to the creation of “Critical Design”: design that addresses the limitations of product design. “Critical design” as a term was coined in 1997 by Anthony Dunne, and it comes from a practice he developed with Fiona Raby when they were research fellows at the Royal College of Art. Critical design as a practice “is one among a growing number of approaches that aim to prevent and define interrogative, discursive, and experimental approaches in design practice and research.”[4] Critical design demands that design stop existing in terms of capitalist production, which is a function of product design, and push product design towards self-examination and cultural critique. Dunne and Raby highlight that by acknowledging that “the design procession needs to mature and find ways of operating outside the tight constraints of servicing industry. At its worst product design simply reinforces capitalist values. Design needs to see this for what it is, just one possibility, and to develop alternative roles for itself. It needs to establish an intellectual stance of its own, or the design profession is destined to lose all intellectual credibility and [be] viewed simply as an agent of capitalism.”[5] By redefining how product design can be created, critical design also creates new ways for an audience to engage with design and understand all of the different facets of design in their daily lives. Feminist Data Set is inspired by this critical lens, taking aspects of the critical design movement and applying it to machine learning.
Similarly, the maker movement helped redefine hardware engineering as a space of exploration and engagement by redefining “engineering.” Engineering went from an expertise held by seasoned computer scientists to a method of investigation and construction that also welcomed everyday tinkerers and explorers. The growing ease and quickness of creating technology moved engineering into a space of play, and with that, into a space where anyone could create, changing what it meant to be “an engineer.” With the advent of the small, affordable, adaptable and easy-to-use Arduinos (microcomputers), the debut of the company Adafruit, the creation of the fair/conference Maker Faire (a gathering for maker enthusiasts), and now with Little Bits (an educational company that creates small toys that teach children about electrical engineering, a pre-step to getting an Arduino), the revolution of the maker movement is similar to the citizen science movement—it’s a technology-for-everyone revolution. When technology components are easy to engage with, able to be both used and remixed, they create a diversity of projects as well as a diversity of community. It’s revolutionary because it creates new spaces but also allows for the examination of processes, like traditional computer science, that once existed in the “walled garden” of the academy. When institutionalized processes are opened up, and become open source, they are re-adapted for an entire community. These processes allow for more input, and one could argue, more equity. But that’s in an ideal world—there are still plenty of issues of racism and sexism, as well as gatekeeping, inside both the open source movement and the maker movement. That being said, the ability to augment, change, remix, or “fork” an experience, technology and code is what makes “making” an important movement in modern world.
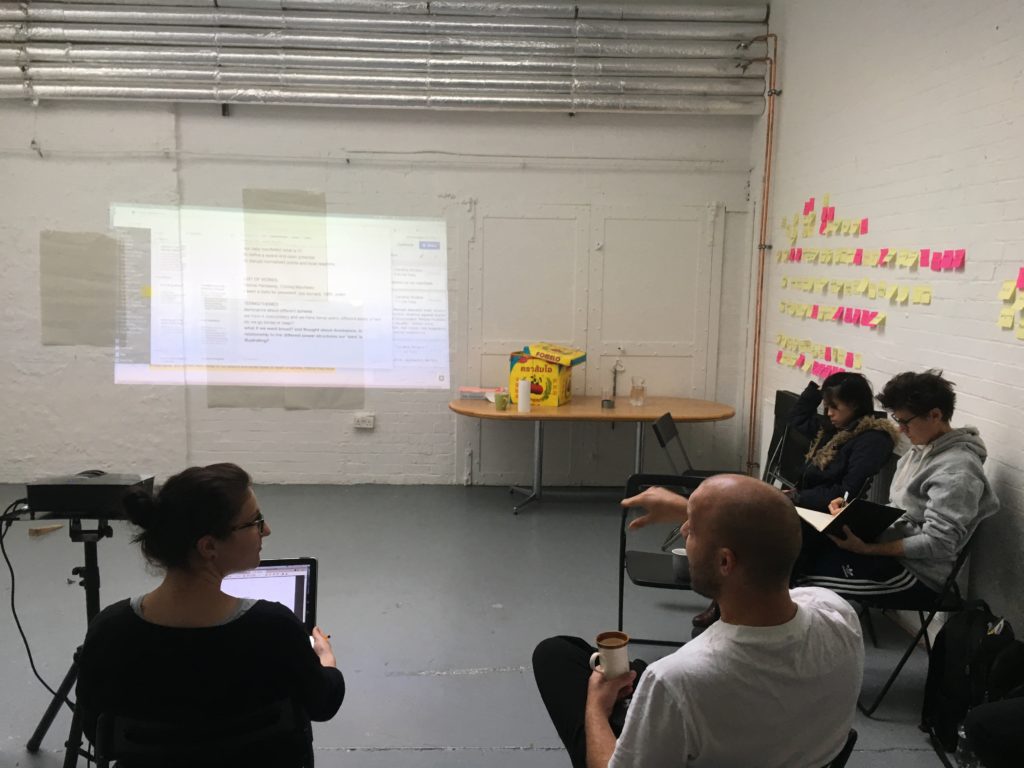
Image of the SPACE Art and Technology workshop; participants are deliberating and labeling the data that’s been submitted to the data set (image courtesy of the artist, Caroline Sinders).
Feminist Data Set operates in a similar vein to Thomas Thwaites’s “Toaster Project,” a critical design project in which Thwaites builds a commercial toaster from scratch, from melting iron ore to building circuits and creating a new plastic toaster body mold. Feminist Data Set, however, takes a critical and artistic view on software and particularly on machine learning. What does it mean to thoughtfully make machine learning, to carefully consider every angle of making, iterating, and designing? Every step of this process needs to be thoroughly re-examined through a feminist lens, and like Thwaites’s toaster, every step has to actually work.
Originally, Feminist Data Set started as collaborative data set built through a series of workshops to address bias in artificial intelligence. The iterative workshops are key; by “slowly” gathering data in physical workshops, we allow a community to define feminism. But I also, workshop by workshop, examine what is in the data set so that I can course correct to address bias. I viewed this as farm-to-table sustainable data set “growing.” Are there too many works by cisgender women or white women in the data set? Then I need to address that in future or follow-up workshops by creating a call for non-cis women, for women of color, and for pieces of work by trans creators.
By design, the project will eventually conclude in creating a feminist AI system. However, there are many steps involved in the process:
- data collection
- data structuring and data training
- creating the data model
- designing a specific algorithm to interpret the data
- questioning whether a new algorithm needs to be created to be ‘feminist’ in its interpretation or understanding of the data and the models
- prototyping the interface
- refining
Every step exists to question and analyze the pipeline of creating using machine learning—is each step feminist, is it intersectional, does each step have bias and how can that bias be removed?
As a way to “design out bias,” I look to the The Critical Engineering Manifesto by Julian Oliver, Gordan Savičić, and Danja Vasiliev. The Critical Engineering Manifesto outlines ten principles as a guide to creating engineering projects, code, systems and ideals. Similar to critical design, it exists to examine the role that engineering and code plays in everyday life, as well as art and creative coding projects. Principles two and seven address the role of shifting technology:
-
The Critical Engineer raises awareness that with each technological advance our techno-political literacy is challenged….
-
The Critical Engineer observes the space between the production and consumption of technology. Acting rapidly to changes in this space, the Critical Engineer serves to expose moments of imbalance and deception.[6]
Similarly, this manifesto (as well as others) helped serve as a basis for writing the original Feminist Data Set Manifesto, created in workshop 0 of the Feminist Data Set in London’s SPACE Art and Technology in October 2017.
The Feminist Data Set Manifesto:
OUR INITIAL INTENTION:
to create a data set that provides a resource that can be used to train an AI to locate feminist and other intersectional ways of thinking across digital media distributed online.OUR INTENTIONS, in Practice, over the course of two days, we created a data set that questions, examines, and explores themes of dominance. Inspired by the cyborg manifesto, our intention to add ambivalence, and to disrupt the unities of truth/information, mediated by algorithmic computation when it comes to expressing power structures in forms of domination, in particularly in relationship to intersectional feminism.
OUR FUTURE INTENTIONS are to create inputs for an artificial intelligence to challenge dominance by engage in new materials and engage with others. We are building, collaboratively a collection.
Through collaboration, we created a new way to augment intelligence and augmented intelligence systems instead focusing on autonomous systems.
OUR MAIN TERMS: disrupt, dominance
MANIFESTO: we are creating a space/thing/data set/capsule/art to question dominance.
This manifesto defined the current and future intentions of the project. Feminist Data Set must be useful, it must disrupt and create new inputs for artificial intelligence, and it must also be a project that focuses on intersectional feminism.
To date, the project has been simply gathering data. The next step in the project will be addressing data training and data collection. In machine learning, when it comes to labeling data and creating a data model for an algorithm, groups will generally use Amazon’s labor force, Mechanical Turk, to label data. Amazon created Mechanical Turk to solve their own machine learning problem of scale: they needed large data sets trained and labeled. Using Mechanical Turk in machine learning projects is standard in the field; it is used everywhere from technology companies to research groups to help label data. For the Feminist Data Set, the question is: Is the Mechanical Turk system feminist? Is using a platform of the gig economy ethical, is it feminist, is it intersectional? A system that creates competition amongst laborers, that discourages a union, that pays pennies per repetitive task, and that creates nameless and hidden labor is not ethical, nor is it feminist.
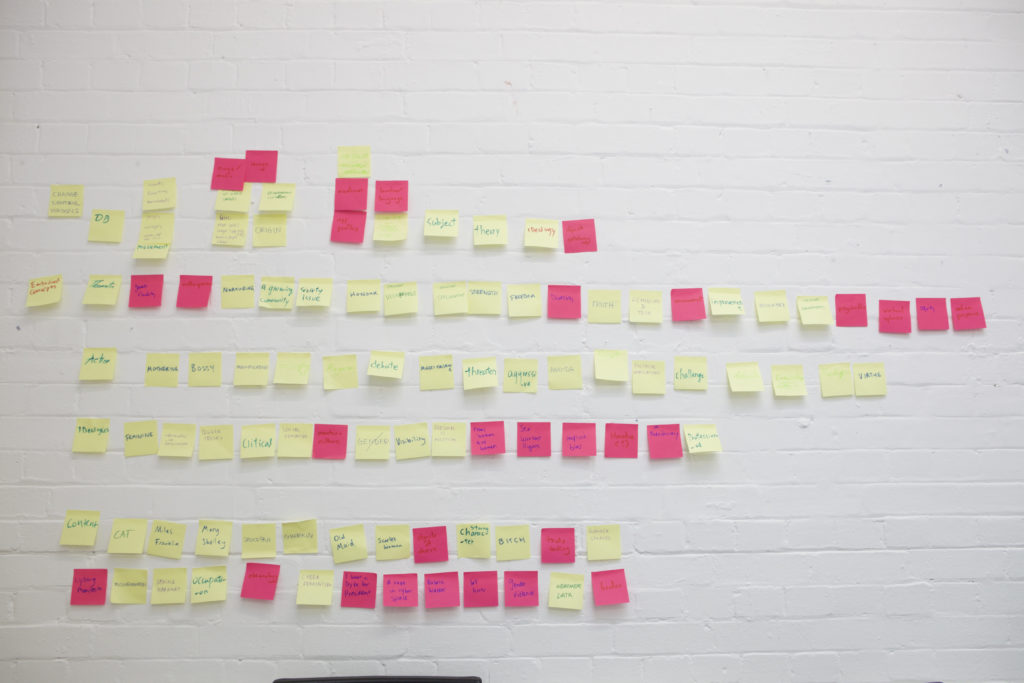
Image of theorizing, planning, and organizing the kinds of data Feminist Data Set would address and occupy during the SPACE Art and Technology workshop (image courtesy of the artist, Caroline Sinders).
In 2019, I will be building, prototyping, and imagining what an ethical mechanical turk system could look like, one created from an intersectional feminist lens that can be used by research groups, individuals, and maybe even companies. This system will be ethical, in the sense that it will allow for more transparency in who trains and labels a data set. The trainers will also be authors, and the system will save data about the data set. This system will also give researchers or project creators the ability to see how much one trainer has trained the data, as well as invite and verify new trainers. Additionally, project creators will be able to pay trainers through this system by suggesting living wage payments. But this system also creates necessary data about the data set itself. This data about the data set includes who labeled or trained it, where are they from, when the data set was “made” or finished, and what’s in the data set (e.g. certain kinds of faces, etc.). If machine learning is going to move forward in terms of transparency and ethics, then how data is trained, how trainers interact with it, and how datasets are used in algorithms and model creation need to be critically examined as well.
Making must be thoughtful and critical in order to create equity. It must be open to feedback and interpretation. We must understand the role of data creation and how systems can use, misuse, and benefit from data. Data must be seen as something created from communities, and as a reflection of that community—data ownership is key. Data’s position inside technology systems is political, it’s activated, and it’s intimate. For there to be equity in machine learning, every aspect of the system needs to be examined, taken apart and put back together. It needs to integrate the realities, contexts, and constraints of all different kinds of people, not just the ones who built the early Web. Technology needs to reflect those who are on the web now.
[1] Tania Bruguera, “Introduction on Useful Art,” April 4, 2011, http://www.taniabruguera.com/cms/528-0-Introduction+on+Useful+Art.htm
[2] “Feminist Principles of the Internet – Version 2.0,” Feminist Principles of the Internet, August 2016, https://www.apc.org/en/pubs/feminist-principles-internet-version-20
[3] “Feminist Principles of the Internet – Version 2.0”
[4] Matt Malpass, Critical Design in Context: History, Theory, and Practices (London: Bloomsbury, 2016), 4
[5] Anthony Dunne and Fiona Raby, Design Noir: The Secret Life of Electronic Objects (August/Birkhauser, 2001), 59.
[6] “The Critical Engineering Manifesto,” The Critical Engineering Working Group (Julian Oliver, Gordan Savičić, and Danja Vasiliev), October 2011-2017, https://criticalengineering.org/
Dilettante Mail
Get updates from us a few times a year.